Engineers Are Helping Draft the Solutions to America's Ills
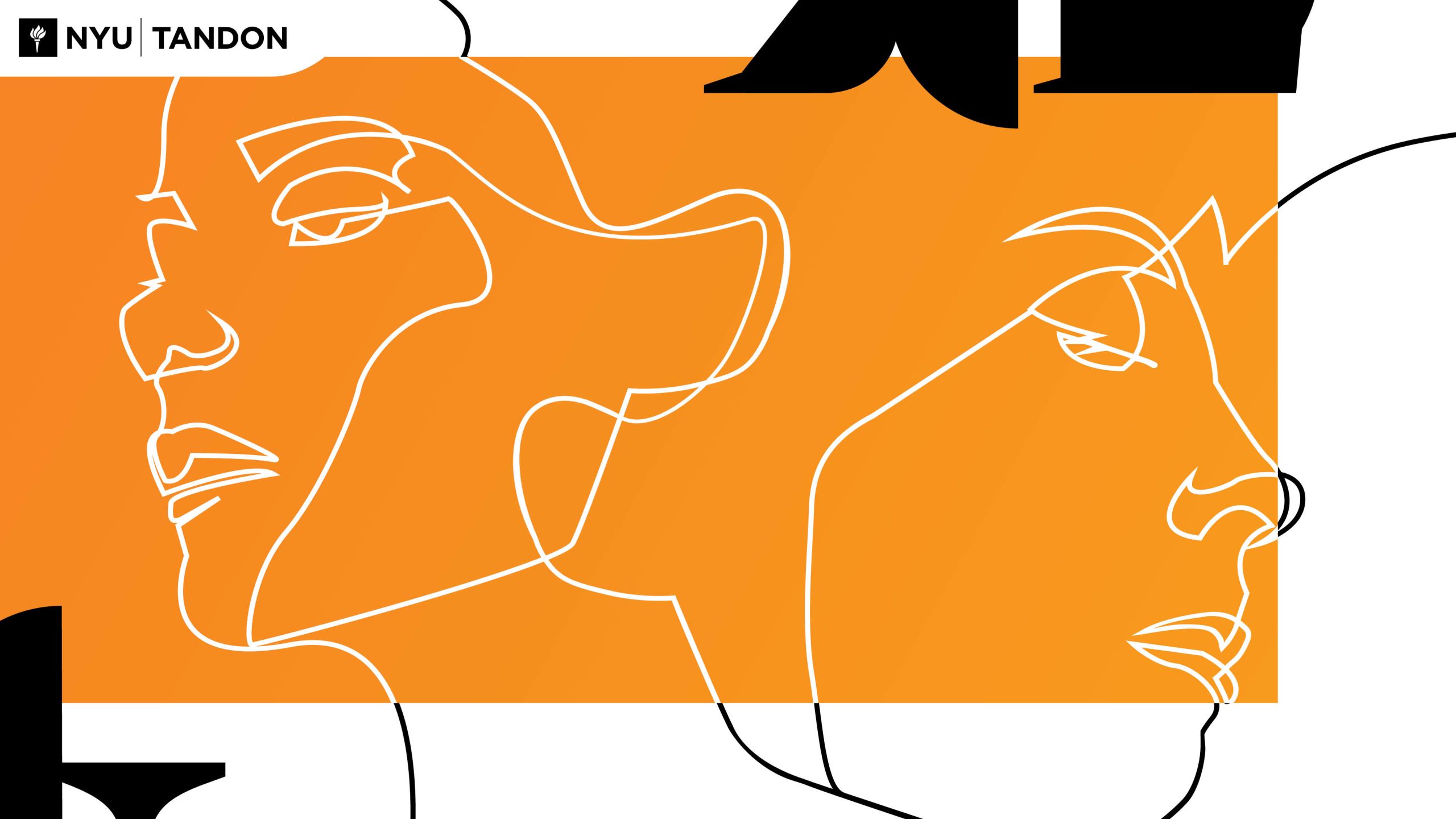
The year 2020 has been one of upheaval. COVID-19 swept through the country, spreading illness and death in a scale we hadn't imagined. Civil unrest exploded in the streets of cities and towns in response to a long history of injustice and police violence. Even expected events like the 2020 election have been marked by chaos and unpredictability.
This was a year that many of the institutions and organizations Americans take for granted began to buckle under the stress of a country in crisis. Political scientists, epidemiologists, activists, and others made their voices heard. But behind the scenes, engineers put their heads down and got to work too. After all, they were used to building things to withstand stress. But this time the project was unconventional — the United States itself. And an unconventional project requires unconventional engineers.
Mapping Disease Transmission Points in Urban Environments
On March 1st — three months after the first reported cases in central China — New York City recorded its first case of COVID-19. In the weeks that followed, the city would become the epicenter of the pandemic in America. For residents, New York became unrecognizable.
Social distancing became the words on everyone’s lips. Limit interactions. Stay inside. Do your duty to fellow citizens. But how would social distancing actually work in a city of over eight million? Engineers in New York began to model the ways humans interact in urban environments, including simple questions no one had really thought about. Like: just how many surfaces do you typically touch on a trip out of the home?
For these engineers, that data needed to be collected. Not just from algorithms, but on the streets. In March, just as New York City began a strict lockdown, a team of 16 student researchers from the New York University Tandon School of Engineering started a project to study individual behaviors outside of COVID-19 hotspots.
Led by Debra Laefer, a professor of civil and urban engineering at NYU Tandon and director of citizen science at the Center for Urban Science and Progress (CUSP), the team captured specific, hyper-local data on the movement and behaviors of people when leaving COVID-19 medical facilities: they created precise records of what people touched, where they went, and whether they were wearing personal protective equipment. Using these records, they identified surfaces and locations most likely to be disease transmission points.
Collectively, the team spent over 1,500 hours collecting data across the city — 5,065 records documenting the behavior of 6,075 individuals around 19 hospitals and urgent care clinics. Over periods of up to 20 minutes, the researchers documented the gender of the subject, objects touched, route taken, and destinations among other factors. The records showed clear trends over time and differences in behavior by gender in both transportation choices and PPE usage.
That data has now been made open and available to researchers studying disease transmission. Laefer predicts that the compendium of real-world data collected by her team will enable researchers to create disease transmission models for cities worldwide — predicting hotspots and allowing public health officials to react quickly in dense locations where quick action can save lives.
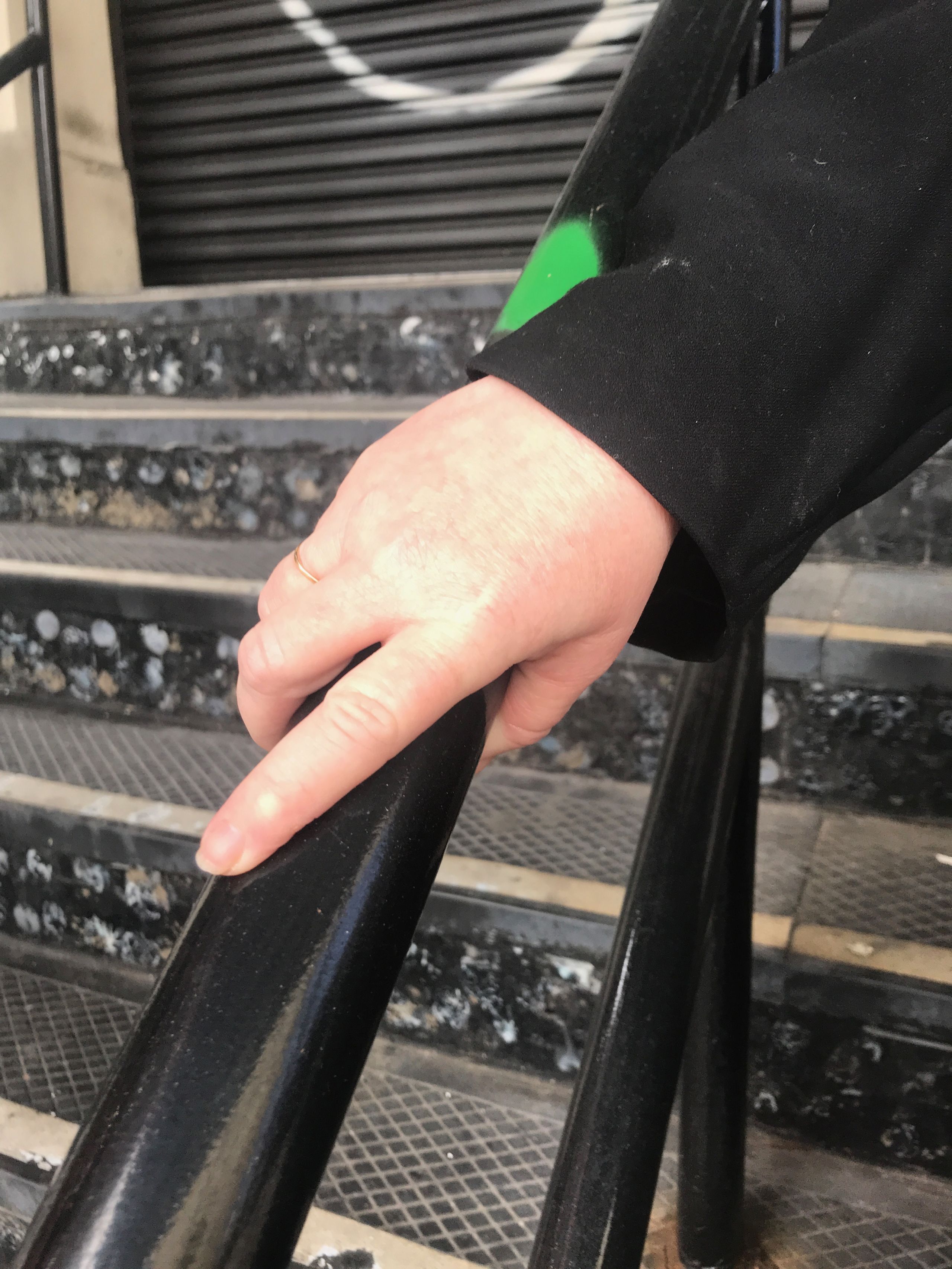
Misinformation and U.S. Elections
Every election has high stakes and contested viewpoints. But over the last 10 years, the realm of public debate has moved almost entirely to social media, where bad actors purposefully spreading misinformation can reach a wide audience. But how can we limit the damage done by blatant misinformation that spreads across Facebook groups and Twitter timelines like wildfire?
During the Democratic primaries, a study conducted by engineers from NYU Tandon revealed that pairing headlines with credibility alerts from fact-checkers, the public, news media, and even AI, can adversely affect the amount a post containing misinformation is shared. However, that effectiveness largely depends on the user’s political alignment and gender.
The study was led by Nasir Memon, professor of computer science and engineering at the New York University Tandon School of Engineering and Sameer Patil, visiting research professor at NYU Tandon and assistant professor in the Luddy School of Informatics, Computing, and Engineering at Indiana University Bloomington. The work involved an online study of around 1,500 individuals to measure the effectiveness among different groups of four so-called “credibility indicators” displayed beneath headlines.
- Fact Checkers: “Multiple fact-checking journalists dispute the credibility of this news”
- News Media: “Major news outlets dispute the credibility of this news”
- Public: “A majority of Americans disputes the credibility of this news”
- AI: “Computer algorithms using AI dispute the credibility of this news”
Official fact-checking sources were overwhelmingly trusted, while the other indicators were much less trusted.
But political alignment strongly affected the result. More than 60% of Democrats were shown to be less likely to share false stories checked by fact checkers, compared to 19 percent of Republicans. Some two percent of Republicans were more likely to share a story rated false by an AI-based fact-checker.
Ultimately, fact-checking was shown to be helpful in slowing the spread of misinformation — depending on who you ask.
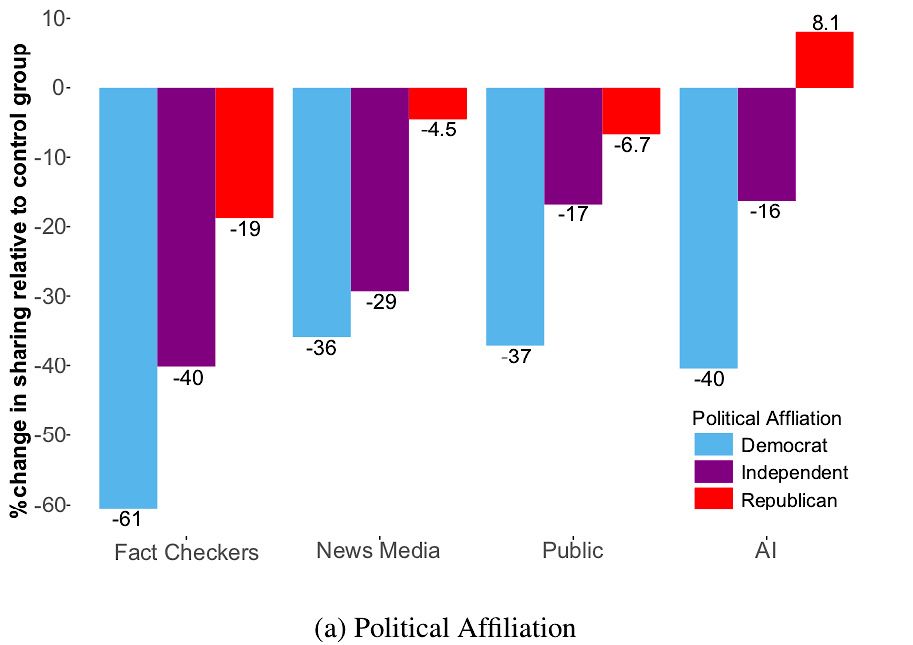
Building an Artificially Intelligent World — Without Bias
This summer, unrest related to police violence gripped the country. Once again, the discrimination and oppression that has marred American history became impossible to ignore.
The data clearly shows that a bias against Black Americans exists — they are more likely to be targeted by law enforcement and less likely to be hired for jobs, to give just two of many examples. Now, both police departments and hiring agencies are increasingly using AI in their work. Theoretically, that could strip out human bias and just focus on the raw data. In practice, the algorithms are just as faulty.
NYU Tandon professor Julia Stoyanovich is trying to fix that. At NYU’s Center for Responsible AI, she explains that because AI systems are dependent on the quality and the representativeness of the data being input, if a system neglects to consider entire groups, it is not likely to perform accurately for those groups. Among other examples:
- Automated decision systems used by employers have been known to discriminate against women in filling tech positions.
- Financial technology has done little to stop mortgage lenders from charging LatinX and Black borrowers higher rates.
- The justice system’s automated methods of predicting recidivism have frequently been shown to be inaccurate.
At the Center, Stoyanovich is applying her research to build open-source tools such as Ranking Facts, a platform that gives users of AI systems easy-to-interpret information on their fairness, stability, and transparency. She and her colleagues are also developing courses on responsible data science and other educational materials (even a comic series — Data, Responsibly — whose first volume is titled “Mirror, Mirror”), so that a new generation of technologists is ready to help build a more just world.
Facebook | Twitter | Instagram | YouTube
This content was paid for and created by New York University. The editorial staff of The Chronicle had no role in its preparation. Find out more about paid content.
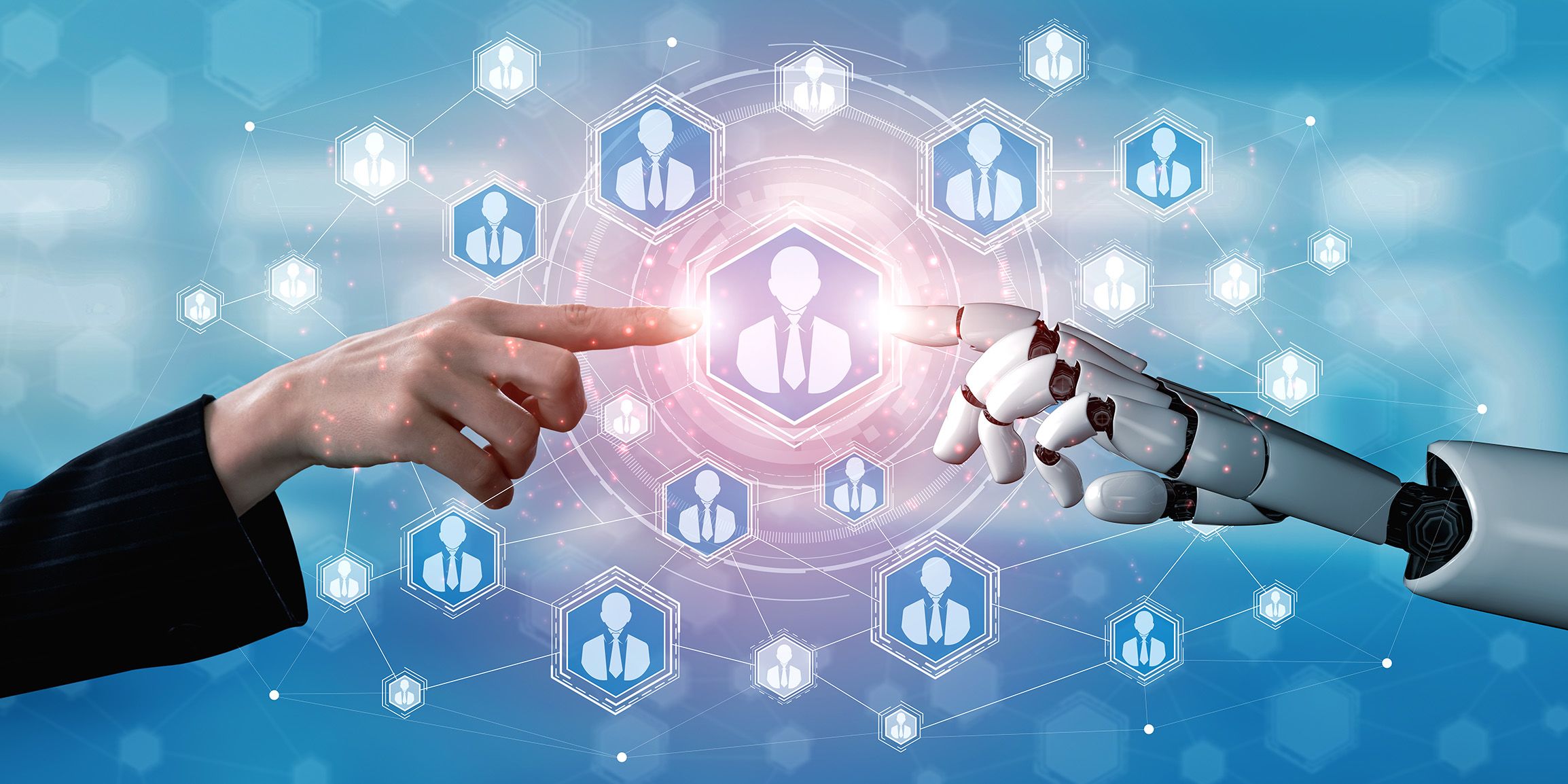